擒数网 (随信APP) | 别人刚刚开始掌握「端到端」技术,但理想智驾已经在不断改进。
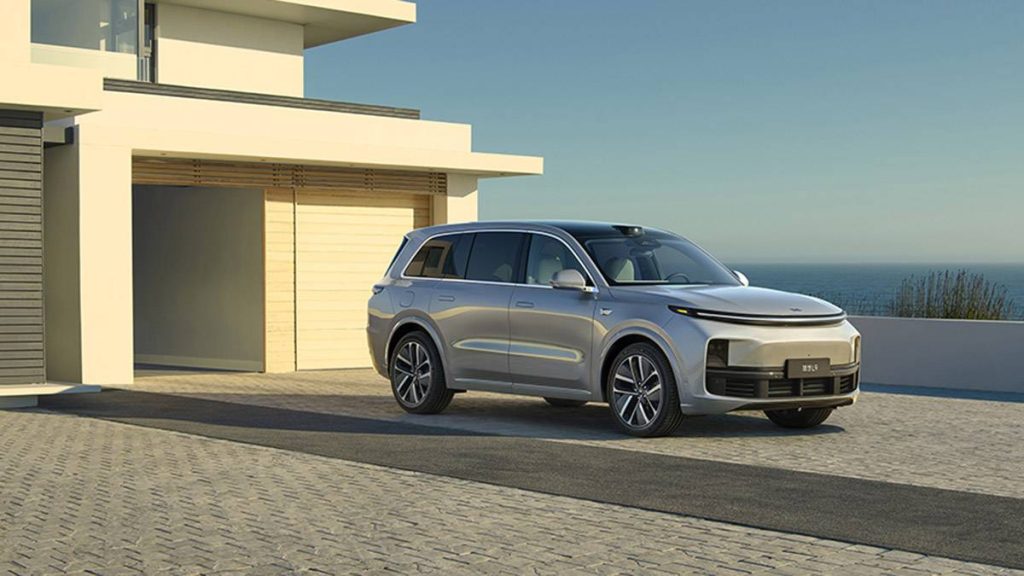
文章目录[隐藏]
擒数网 (随信APP) | 别人刚刚开始掌握「端到端」技术,但理想智驾已经在不断改进。
【微信/公众号/视频号/抖音/小红书/快手/bilibili/微博/知乎/今日头条同步报道】
自动驾驶技术发展了这么多年,最大的变化是什么?
在写下这篇文章的前一天,两位汽车行业朋友来到爱范儿,和我们坐下聊了聊。聊到的内容有很多,从产品推广到行业趣闻,而自动驾驶作为行业热议的一个分支,自然也成为了我们讨论的焦点之一。
回顾自动驾驶这些年来的发展,变化其实有不少,包括传感器的迭代、车端算力的提升、从高精地图过渡到占用网络等。但在这些变化中,最引人注目的突破当属大模型的加入。
大模型,让自动驾驶技术的应用,变得触手可及。
10 月 23 日,理想汽车全新一代双系统智能驾驶解决方案「端到端+VLM」正式开始全量推送,理想汽车的智能驾驶,从此步入了 AI 大模型的时代。
像人一样思考,像人一样驾驶,如今的理想汽车,正在实现这一愿景。
好不容易搞懂了端到端,VLM 又是什么?
关于端到端到底是什么?是从哪个「端」到哪个「端」?别说普通消费者了,就连不少媒体从业者都没有搞清楚。
不少厂商都曾对此做出过解释,其中解释得最通俗易懂的,还是理想汽车:
一端,是传感器:摄像头、激光雷达等传感器,它们就像是人的眼睛,负责输入环境信息。另一端,是行驶轨迹:接收了来自传感器的信息后,系统会输出「动态障碍物」、「道路结构」、「占用网络 Occ」和「规划轨迹」。一直以来,无论是主机厂还是自动驾驶企业,都在不断宣传自家的智驾系统有多么类人,多么像「老司机」。然而,一些「老司机」们习以为常的场景,在很长一段时间里,都是难以解决的行业难题。最典型的就是环岛这一场景,因为场景复杂、感知受限,因此在今年 7 月之前,还没有几家车企能够实现「老司机」般的进出环岛。
理想智驾技术研发负责人贾鹏曾对爱范儿和董车会表示,对于感知和规控分离的分段式智驾方案来说,在环岛场景里,感知模型需要为规控模型做「各种各样的假设。」一体式的端到端方案则不同,其具备更强的复杂道路结构的理解能力,可以运用人类驾驶员数据训练出不同的环岛类型、不同出入口的进出轨迹,自主选择合适的行进路线。如此一来,原有的道路拓扑和人工定义的规则,就再是必须的了。
关于环岛这件事,贾鹏还分享过一个「好玩的故事」。在我们(的模型数据包含)大概 80 万 clips(视频片段)的时候,还过不了环岛,后来突然有一天发现我们(喂了)100 万 Clips(之后)它自己能过环岛,我觉得是 100 万(视频片段)里头刚好有一些环岛数据放在里面了。「模型确实很厉害,」贾鹏补充道,「你喂了什么数据他就能学会,这是模型的魅力所在。」
理想如今推出的全量版本基于 V4.8.6 模型,后者是在 400 万 clips 的基础上迭代的第 16 个版本。和以往相比,新模型对于超车场景和导航信息的理解能力得到提升,同时,障碍物的检测更加精准,绕行的幅度也更为合理。
因此不仅是环岛,像 U 型掉头、拥堵时的蠕行和博弈、十字路口等传统复杂场景,如今的「端到端+VLM」智驾系统,都能够很好地自主处理,甚至还支持 P 档激活——在路边停车时,用户原地双击拨杆来激活智驾系统,不必再像以前一样,必须在车道内才能激活。介绍完端到端模型的能力,接下来就是 VLM 模型。
VLM 模型是一种视觉语言模型,理想是第一个将视觉语言模型成功部署在车端芯片的厂商,使自动驾驶具备了未知场景的逻辑思考能力。
也就是说,它能够像人一样思考。
举个例子,能够生成行驶轨迹的端到端模型,完全具备通过收费站的能力,但它在面对收费站时,并不是很清楚自己应该走哪条道,最后只能随便挑一条来走。而 VLM 模型,则能够像人类一样理解物理世界的复杂交通环境和中文语义,可以清楚地分辨 ETC 车道和人工车道,并辅助端到端模型做出正确的决策。类似的场景其实还有很多,如公交车道和潮汐车道的识别、学校路段等路牌的识别、主辅路的进出等。不仅如此,在遇到施工场景、坑洼路面甚至是减速带时,VLM 模型也能很好地理解,进行提醒和降速。截至目前,理想汽车的 VLM 视觉语言模型已经拥有了 22 亿的参数量,对物理世界的复杂交通环境具有更拟人的理解能力。此外,在 OTA 6.4 版本中,高速 NOA 功能也得到了优化,在高速 &城市快速路场景中,系统可以更早地识别前方慢车,超车动作更加高效安全。总而言之,在端到端+VLM 双系统的帮助下,如今面向用户的 OTA 6.4,其拟人化程度上到了一个新的台阶。
理想的「快」与「慢」
从技术架构来看,理想汽车这两年经历了三次比较大的调整。从需要先验信息的 NPN 网络,再到基于 BEV 和占用网络的无图 NOA,再到如今的一体化端到端技术路线。第一代 NPN 架构比较复杂,包含了感知、定位、规划、导航、NPN 等模块,它们共同支撑起了理想汽车当时 100 城的城市 NOA 推送。第二代无图 NOA,理想汽车引入了端到端大模型,模块数量大幅缩减,只剩下了感知和规划,不再需要等待先验信息的更新。理想的这一步,让车企的「卷」,不再局限于无聊的开城数量,真正实现了有导航就能开。今年 5 月,理想汽车招募了 1000 位用户,正式开启了无图 NOA,也就是 AD Max 3.0 的公测。当时的用户反馈,远远超出了理想汽车的预期,短短两个月后,理想汽车就为 24 万多位理想 AD Max 用户推送了这次升级。只不过,这个时候的端到端,还是一个分段式的端到端,第三代智驾方案,才是真正意义上的一体式端到端——从输入到输出,全部由一个模型实现,中间没有任何规则的参与。在以往,无论是有图方案还是无图方案,都依赖工程师根据各种各样的道路场景去编写规则,力图穷举所有道路状况和与之对应的方案,让智驾的范围尽可能地广。通常来说,厂商会把场景大致分为三种:高速场景、城区场景和泊车场景。这几大场景又可以继续细分,规控工程师们则需要针对这些场景来编写代码。但面对错综复杂的现实世界,这样的做法显然不够现实。而一体式端到端,则可以学习人类开车的过程,接收传感器信息后,直接输出行驶轨迹。有没有发现,这个时候,提升智驾能力最重要的因素,从工程师变成了数据。而理想,最不缺的就是数据。10 月 14 日,理想汽车迎来了第 100 万辆整车在江苏省常州基地下线,中国首个百万辆新势力车企就此诞生。根据理想汽车公布的数据,在 30 万元以上的理想车型中,AD Max 用户的比例,高达 70%——每过一个月,这些车都能给理想提供十几亿公里的训练数据。另外,理想很早就意识到数据的重要意义,打造了关于数据的工具链等基础能力,比如理想的后台数据库实现了一段话查找当时,写一句「雨天红灯停止线附近打伞路过的行人」,就能找到相应的数据。正是凭借庞大的训练数据和完善的控制链,理想智驾实现了在行业中的「后来居上」,用端到端和 VLM 组成了自己的「快」与「慢」。在理想看来,这套双系统智驾方案,类似于诺贝尔奖获得者丹尼尔·卡尼曼在《思考,快与慢》中的快慢系统理论:人的快系统依靠直觉和本能,在 95% 的场景下保持高效率;人的慢系统依靠有意识的分析和思考,介绍 5% 场景的高上限。其中,端到端是那个「快系统」,而 VLM 自然就是「慢系统」了。
理想同学,我要去这里
除了智能驾驶方面的升级,OTA 6.4 在用户交互方面也引来了革新。这里同样分为「快」和「慢」两个部分。
作为「快系统」的端到端模型所对应的通常为文字弹窗,为驾驶员实时提供导航、交规、效率、博弈等执行逻辑和动作。
对于「慢系统」VLM 视觉语言模型,理想则为它准备了全新的图文视窗。在特殊场景下,将前方感知到的画面投射到页面内,配合文案讲解模型的思考过程和结果。
在文字弹窗和图文视窗的配合下,无论系统执行何种车控动作,驾驶员都能提前知悉。对于那些初次体验智驾的消费者来说,这种直观的信息展示也有助于迅速建立他们对智能驾驶系统的信任感。不得不承认,理想汽车对用户需求的理解确实非常精准。
在我们对于未来的畅想中,智驾和智舱总是绑定在一起的,在 OTA 6.4 版本中,理想也为它的智能空间带来了不少升级。
首先是新增的任务大师 2.0 全面接入了理想同学和 Mind GPT 的能力,在大模型的加持下,任务大师的表现更为智能。
Mind GPT 加持下的理想同学,不仅能够在周末家庭短途旅行和解答日常小疑问这两个场景里发挥作用,结合新升级的高德 AutoSDK 750 版本导航地图,理想同学可以通过「触控+语音」的方式,让驾驶员迅速进行目的地搜索。比如说,指着地图上的某个位置,让它帮你搜索充电站任一品牌的充电桩,甚至还可以指定功率。总之,全新的理想同学完全可以让你不必拿起手机,你可以用最自然直观的方式,轻松设置导航路径。端到端负责驾驶,VLM 替你思考,而你只需简单地指引方向。
英文版:
What is the biggest change in the development of autonomous driving technology over the years?
On the day before writing this article, two friends from the automotive industry came to iFanr and sat down to chat with us. We discussed a variety of topics, from product promotion to industry anecdotes, and autonomous driving, as a branch of the industry that is widely discussed, naturally became one of our focal points.
Looking back on the development of autonomous driving in recent years, there have been quite a few changes, including iterations of sensors, improvement in on-board computing power, transitioning from high-definition maps to network occupancy, and more. But among these changes, the most noteworthy breakthrough is undoubtedly the introduction of large models.
Large models make the application of autonomous driving technology more accessible.
On October 23, Ideal Auto's new generation dual-system intelligent driving solution "End-to-End+VLM" officially began full-scale deployment, marking the era of AI large models for Ideal Auto's intelligent driving.
Thinking like a human, driving like a human, that's what Ideal Auto is achieving now.
Just understood End-to-End, what is VLM?
What exactly is End-to-End? From which "end" to which "end"? Even many media practitioners have not figured it out.
Many manufacturers have previously explained this, with the most simple and easy to understand explanation coming from Ideal Auto:
One end is sensors: cameras, LiDAR sensors, etc., they are like human eyes, responsible for inputting environmental information. In addition, there are specially designed input information, such as the vehicle's position, posture, navigation, etc.
The other end is the driving trajectory: after receiving information from the sensors, the system outputs "dynamic obstacles," "road structure," "occupancy network Occ," and "planned trajectory." The first three perceptual tasks are mainly presented to users through the screen, and the fourth "driving trajectory" is what we ultimately need to map from the sensors.
▲ Ideal Drive End-to-End Architecture Diagram
It is easy to see that the process from receiving information from sensors to the system outputting a driving trajectory is very similar to us driving ourselves—our eyes are responsible for receiving information, and our hands naturally steer the steering wheel to guide the vehicle onto the correct trajectory.
Yes, relying on the End-to-End model, Ideal's new generation intelligent driving system is driving like a human.
All along, whether it is a mainstream manufacturer or an autonomous driving company, they have been constantly promoting how human-like their intelligent driving systems are, how much they resemble "experienced drivers." However, some scenarios that "experienced drivers" are accustomed to have been industry challenges that were difficult to solve for a long time.
The most typical one is the roundabout scenario, because of its complexity and limited perception, until July of this year, there were not many car manufacturers that could achieve the same level as an "experienced driver" entering and exiting a roundabout.
Jia Peng, the head of Ideal's intelligent driving technology research and development, once told iFanr and Dong Chehui that for the segmented intelligent driving solutions with separated perception and control, in the roundabout scenario, the perception model needs to make "various assumptions" for the control model.
For example, making a U-turn, you also need to fit the U-turn line. Different roundabouts have different U-turns, different curvatures, so you'll find it's hard to have a set of code that can handle all roundabout U-turns, there are too many types.
In contrast, the integrated End-to-End solution is different, as it has a stronger ability to understand complex road structures, can train out different types of roundabouts, different entry and exit trajectories using human driver data, and autonomously choose the appropriate driving route.
As a result, the original road topology and artificially defined rules are no longer necessary.
Regarding the roundabout scenario, Jia Peng also shared a "fun story."
When we (the model data pack) had about 800,000 clips (video clips), we still couldn't get through the roundabout. Then one day suddenly we found that after we (fed) 1,000,000 clips, the model was able to navigate the roundabout itself. I think within those 1,000,000 clips, there happened to be some roundabout data included.
"The model is really amazing," Jia Peng added, "it can learn whatever data you feed it, that's the charm of the model."
The full version launched by Ideal now is based on the V4.8.6 model, the 16th iteration based on 4 million clips. Compared to the past, the new model has improved understanding capabilities for overtaking scenarios and navigation information. Additionally, obstacle detection is more precise, and the detour is more rational.
Therefore, not only roundabouts, but also traditional complex scenarios such as U-turns, crawling and negotiations in congestion, intersections, etc., are all well handled by the "End-to-End+VLM" intelligent driving system. It even supports P-gear activation—
When parking on the side of the road, users can double-click the lever in place to activate the intelligent driving system, no longer needing to be in the lane to activate it as before.
After introducing the capabilities of the End-to-End model, next is the VLM model.
The VLM model is a visual language model, and Ideal is the first manufacturer to successfully deploy a visual language model on a vehicle chip, giving autonomous driving the ability to logically think about unknown scenarios.
In other words, it can think like a human.
For example, the End-to-End model that can generate driving trajectories fully has the ability to pass through toll stations, but when it faces a toll station, it is not very clear which lane it should take, and ends up randomly choosing one.
The VLM model, on the other hand, can understand complex physical traffic environments and Chinese semantic meanings like a human, clearly distinguishing ETC lanes from manual lanes and assisting the End-to-End model in making the correct decisions.
There are many similar scenarios, such as recognizing bus lanes and tidal lanes, identifying school road signs, entering and exiting main and auxiliary roads, and more. Additionally, when encountering construction scenes, potholed roads, or speed bumps, the VLM model can understand well and provide reminders and slow down.
As of now, Ideal Auto's VLM visual language model has 2.2 billion parameters, with a better understanding of the complex traffic environment in the physical world.
Furthermore, in the OTA 6.4 version, the high-speed NOA function has also been optimized. In high-speed and urban expressway scenarios, the system can identify slow vehicles ahead earlier, making overtaking actions more efficient and safe.
Overall, with the help of the End-to-End+VLM dual system, the user-facing OTA 6.4 version today has reached a new level of humanization.
Ideal's "Fast" and "Slow"
From a technical architecture perspective, Ideal Auto has undergone three significant adjustments in the past two years.
From the NPN network that required prior information, to the graph-free NOA based on BEV and network occupancy, to the integrated end-to-end technology route today.
The first-generation NPN architecture was quite complex, including perception, localization, planning, navigation, NPN modules, etc., supporting Ideal Auto's city NOA push in 100 cities at that time.
The second generation graph-free NOA introduced by Ideal Auto brought in the end-to-end large model, significantly reducing the number of modules to just perception and planning, no longer waiting for prior information updates.
With this step, Ideal Auto's "roll-up" is no longer limited to boring opening city numbers but truly enabling driving with navigation.
In May of this year, Ideal Auto recruited 1,000 users and officially launched the graph-free NOA, which is the AD Max 3.0 beta. The user feedback at that time far exceeded Ideal Auto's expectations. Within just two months, Ideal Auto had pushed out this upgrade to over 240,000 AD Max users.
However, at this point, the end-to-end was still a segmented end-to-end system. The third-generation intelligent driving solution is the truly integrated end-to-end system—from input to output, all done by a single model, with no rules involved in the middle.
In the past, whether it was a graph scheme or a graph-free scheme, engineers would need to write rules based on various road scenarios to exhaust all road conditions and corresponding solutions in the intelligent driving scope.
Generally, manufacturers would roughly categorize scenes into three types: high-speed scenarios, urban scenarios, and parking scenarios. These major scenarios could be further subdivided, and control engineers would need to write code for these scenarios.
But faced with the complexity of the real world, this approach is clearly not realistic. The integrated end-to-end system can learn the process of human driving, receive sensor data, and directly output driving trajectories.
Have you noticed that at this point, the most important factor in improving intelligent driving capabilities has shifted from engineers to data? And Ideal has no shortage of data.
On October 14, Ideal Auto celebrated the production of its one millionth vehicle at its base in Changzhou, Jiangsu Province, marking the birth of China's first new energy vehicle manufacturer to produce one million vehicles. According to Ideal Auto's data, in Ideal models priced above 300,000 RMB, the proportion of AD Max users is as high as 70%—
Every month, these vehicles provide Ideal with billions of kilometers of training data.
In addition, Ideal realized the importance of data early on and built basic capabilities around data, such as an internal database where typing a sentence like "a pedestrian with an umbrella passing by near the red light stop line on a rainy day" would bring up relevant data.
With a vast amount of training data and a well-developed control chain, Ideal Drive has achieved a "latecomer's advantage" in the industry, combining end-to-end and VLM to create its own "fast" and "slow."
In Ideal's view, this dual-system intelligent driving solution is similar to psychologist Daniel Kahneman's theory of fast and slow systems in "Thinking, Fast and Slow":
The human fast system relies on intuition and instinct, maintaining high efficiency in 95% of scenarios; the human slow system relies on conscious analysis and thought, raising the upper limit in 5% of scenarios.
Here, the End-to-End is the "fast system," while VLM naturally becomes the "slow system."
Langyan Peng believes that whether an autonomous driving system is at level L3 or L4 ultimately depends on the VLM model, which is the key to addressing unknown scenarios and enhancing the upper limit of capability.
"Ideal, I want to go here"
In addition to upgrades in intelligent driving, OTA 6.4 also brought innovation in user interaction.
Here, it is also divided into two parts: "fast" and "slow."
For the "fast system" corresponding to the End-to-End model, typically it displays text pop-ups that provide real-time navigation, traffic regulations, efficiency, and negotiation logic and actions to the driver.
For the "slow system" VLM visual language model, Ideal has prepared a brand new graphic-text window. In special scenarios, projected images of the perceived front scene are displayed on the page, with accompanying text explaining the model's thought process and results.
With the combination of text pop-ups and graphic-text windows, no matter what vehicle control action the system takes, the driver can be aware in advance. For consumers experiencing intelligent driving for the first time, this intuitive display of information helps quickly build their trust in the intelligent driving system.
It must be acknowledged, Ideal Auto's understanding of user needs is indeed very accurate.
In our envisioning of the future, intelligent driving and intelligent cabin are always linked. In the OTA 6.4 version, Ideal has also brought many upgrades to its intelligent space.
Firstly, the newly added Task Master 2.0 fully integrates the capabilities of Ideal Companion and Mind GPT. With the support of the large model, Task Master's performance is more intelligent.
With Mind GPT, Ideal Companion can now be used in weekend family trips and daily small query scenarios. Combined with the upgraded Amap AutoSDK 750 version of the navigation map, Ideal Companion can quickly search for destinations through "touch + voice," allowing drivers to set navigation paths efficiently.
For example, pointing to a location on the map and instructing it to search for any brand of charging station, or even specifying the power. In short, the brand new Ideal Companion allows you to set navigation routes effortlessly without having to pick up your phone, using the most natural and intuitive method.
The End-to-End handles the driving, the VLM thinks for you, and all you have to do is give simple directions.
别人刚上「端到端」,理想智驾却又迭代了
#别人刚上端到端理想智驾却又迭代了
关注流程:打开随信App→搜索擒数网随信号:973641 →订阅即可!
公众号:擒数网 抖音:擒数网
视频号:擒数网 快手:擒数网
小红书:擒数网 随信:擒数网
百家号:擒数网 B站:擒数网
知乎:擒数网 微博:擒数网
UC头条:擒数网 搜狐号:擒数网
趣头条:擒数网 虎嗅:擒数网
腾讯新闻:擒数网 网易号:擒数网
36氪:擒数网 钛媒体:擒数网
今日头条:擒数网 西瓜视频:擒数网